Artificial Intelligence (AI) is revolutionizing the manufacturing industry, but it’s not without its challenges. As someone deeply immersed in the world of AI, I’ve witnessed firsthand the hurdles that companies face when implementing AI technologies in their manufacturing processes. From data security concerns to workforce reskilling, navigating these obstacles is crucial for the industry’s advancement.
Despite the challenges, the future of AI in manufacturing looks promising. As I delve into the latest trends and innovations shaping the landscape, it’s clear that AI is set to enhance efficiency, productivity, and decision-making in ways we’ve never seen before.
Artificial Intelligence in Manufacturing
Data security remains a top concern in AI implementation within the manufacturing industry. As data becomes more accessible and utilized, protecting sensitive information becomes paramount.
- Cyberattacks pose a significant threat to AI systems, potentially compromising manufacturing data and operations. Implementing robust security measures is crucial to safeguard against breaches.
- Compliance regulations, such as GDPR and CCPA, add complexity to AI implementation, requiring manufacturers to navigate legal frameworks to ensure data privacy.
- Data encryption and anonymization techniques play a vital role in protecting data, minimizing the risk of unauthorized access and data breaches.
- Regular audits and security assessments are essential to identify vulnerabilities and strengthen defenses in AI-driven systems.
Addressing data security concerns is fundamental to fostering trust in AI technologies and ensuring sustainable growth in the manufacturing sector.
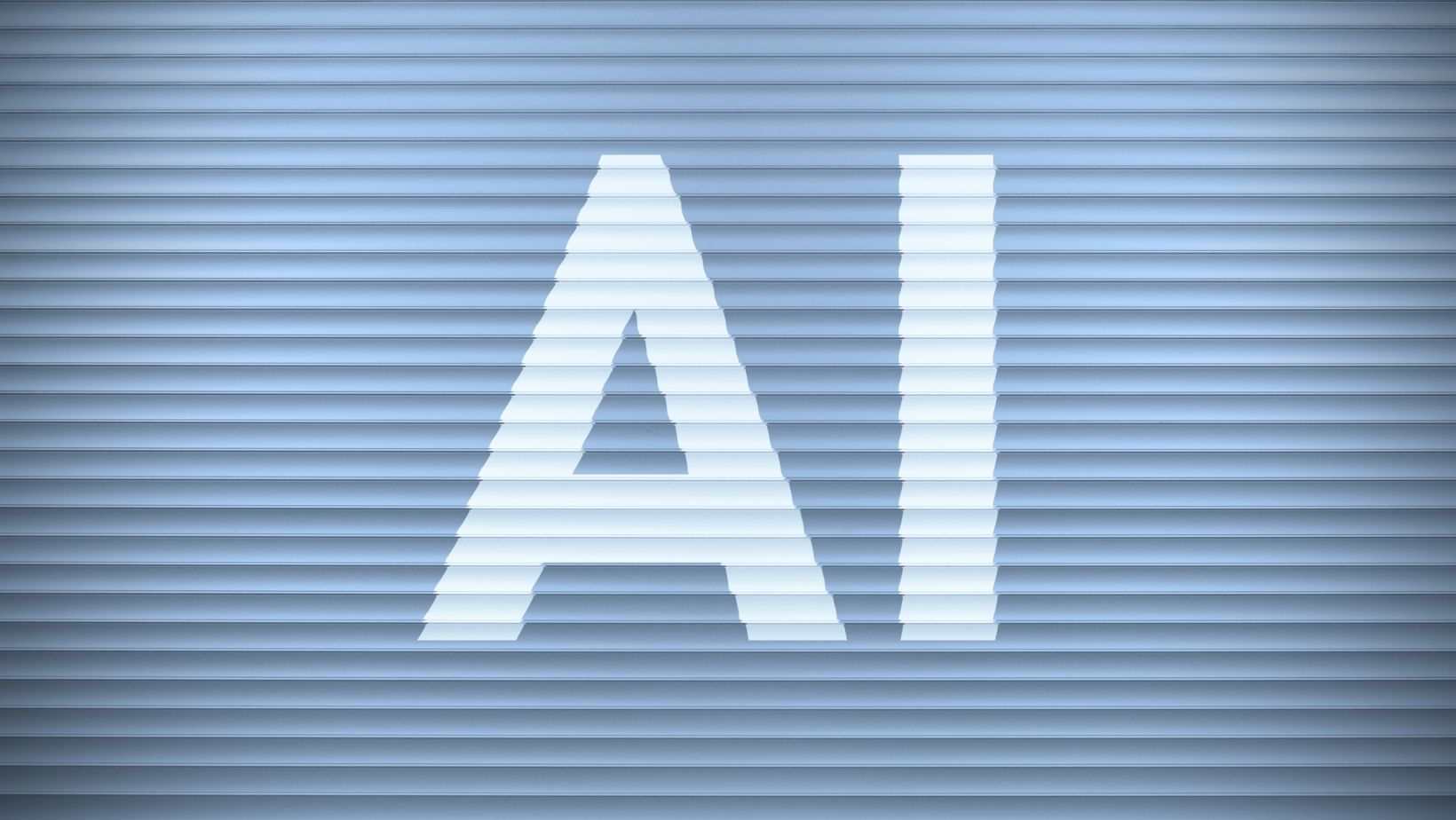
Workforce Reskilling for AI Integration
Incorporating Artificial Intelligence (AI) into manufacturing processes necessitates a workforce equipped with the skills to operate, maintain, and optimize AI technologies. As automation and AI continue to augment traditional manufacturing methods, the need for upskilling and reskilling employees becomes paramount.
Addressing the AI Skill Gap
- Training programs should focus on AI fundamentals, data analysis, and programming languages to empower employees with the necessary know-how to navigate AI systems.
- Investment in continuous learning opportunities ensures that the workforce remains agile and adept at leveraging AI tools for enhanced productivity.
The Role of Upskilling in Innovation
- Upskilling programs foster a culture of innovation within manufacturing organizations, encouraging employees to experiment with AI applications and brainstorm creative solutions.
- Equipped with AI proficiency, the workforce can drive efficiency, optimize workflows, and uncover new avenues for growth in a rapidly evolving industry.
- Embracing collaborative learning environments and mentoring programs promotes knowledge sharing and accelerates skill development across all levels of the organization.
- Leveraging AI-driven platforms for personalized learning experiences tailors training to individual needs, maximizing the potential for skills acquisition and retention.
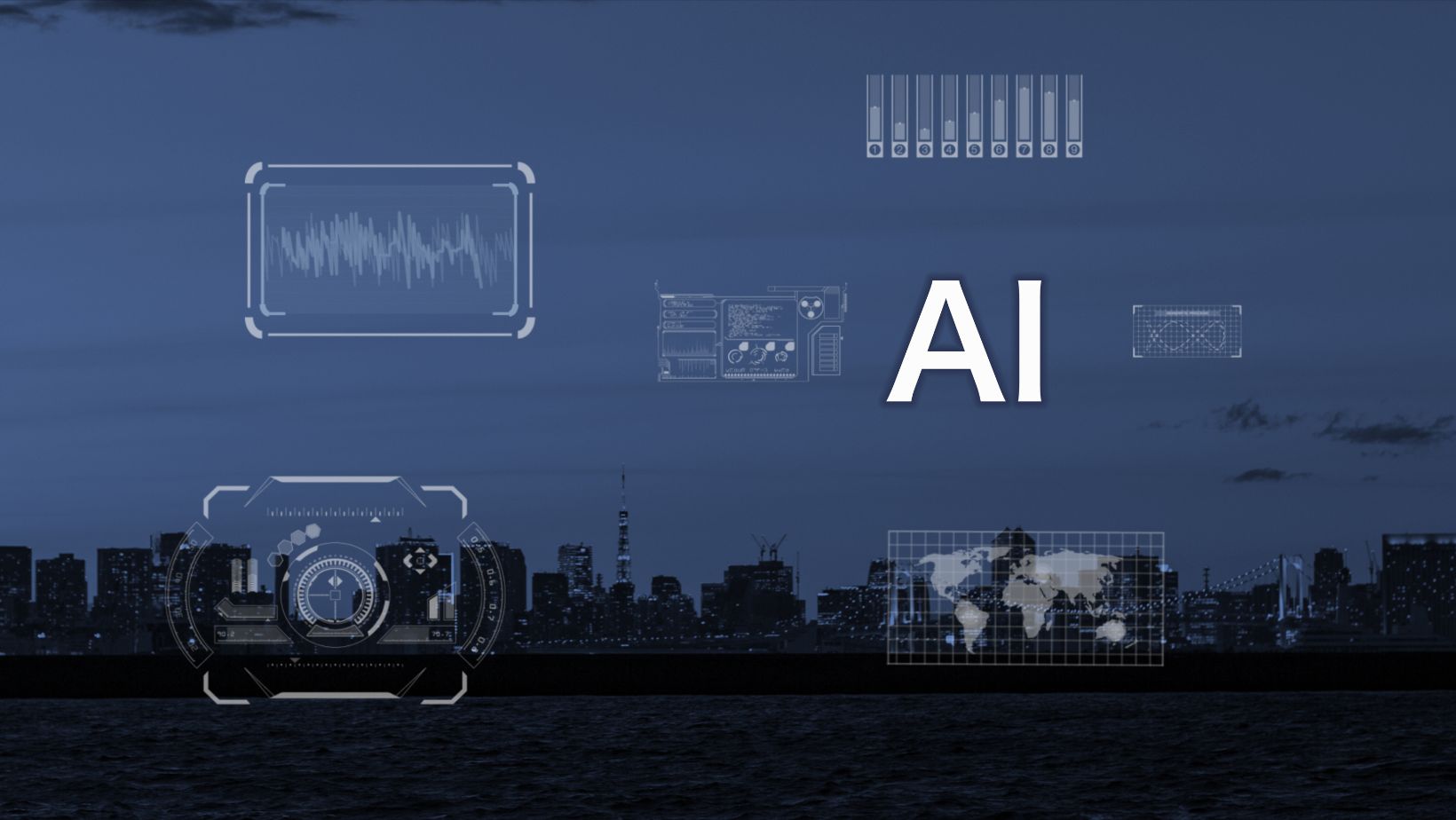
Overcoming Technical Hurdles in Manufacturing AI
When delving into the realm of Artificial Intelligence (AI) in manufacturing, one cannot overlook the technical challenges that may arise. As technology continues to advance, ensuring the seamless integration of AI solutions becomes paramount for industry leaders like myself.
Here are some key strategies for overcoming technical hurdles in manufacturing AI:
- Data Quality: Accurate and relevant data is the lifeblood of AI systems. Addressing data quality issues through data cleansing and standardization is essential for optimal AI performance.
- Algorithm Selection: Choosing the right algorithms for specific AI applications is crucial. Understanding the nuances of different algorithms and their suitability for manufacturing processes is key to enhancing efficiency.
- Model Interpretability: Ensuring that AI models are interpretable is vital for gaining insights into manufacturing operations. Explainable AI techniques can help in interpreting model decisions and building trust in AI systems.
- Scalability: As manufacturing operations expand, AI solutions must be able to scale effectively. Designing scalable AI architectures and implementing robust infrastructure are essential for meeting growing demands.
- Cybersecurity: Safeguarding AI systems from cyber threats is a top priority. Implementing robust security measures, such as encryption and access controls, is crucial for protecting sensitive manufacturing data.
Promising Trends in AI for the Manufacturing Industry
As I delve into the world of AI in manufacturing, I can’t help but notice the exciting trends shaping the future of the industry. Here are some developments that hold significant promise:
- Predictive Maintenance: AI algorithms can analyze equipment sensor data to predict potential breakdowns, enabling proactive maintenance and minimizing costly downtime.
- AI-Driven Quality Control: By leveraging AI for image analysis and defect detection, manufacturers can ensure product quality standards are met consistently.
- Supply Chain Optimization: AI algorithms optimize supply chains by forecasting demand, improving inventory management, and enhancing overall operational efficiency.
Incorporating Machine Learning models enhances decision-making processes, enabling manufacturers to make data-driven choices for improved outcomes. The application of AI-driven Robotics streamlines production lines, enhancing productivity and precision in manufacturing processes. Some organizations are usingĀ elements of photography to make images more interesting and interim while ensuring quality.