Payment fraud is a massive issue.
Losses from online fraudulent payments are predicted to amount to $343 billion for merchants between 2023 and 2027. How can you comprehend those numbers? And in 2023, 80% of businesses were victims of payment fraud attacks/attempts. Even if they were minor or it was only an attempt, that’s almost all organizations.
Despite the advancements that have been made recently, frauds and a high number of payment frauds tend to occur frequently. Below, we’ll look at how machine learning and artificial intelligence (AI) could be the answer.
Powerful Machine Learning Algorithms
Machine learning has become the center of recently established transaction fraud detection systems with unprecedented power to analyze multidimensional data. ML algorithms are not limited by preset rules like their predecessors, and they can learn acceptable standards from vast amounts of historical data.
- Supervised Learning: Average machine algorithms are restricted by the standard training data. Advanced MLs can study labeled data sets consisting of legitimate and scam transactions and learn the difference, eventually being able to spot fraudulent patterns for that instant scenario.
- Unsupervised Learning: If there’s no demonstrable evidence of fraud, novel or evolving risks can be analyzed using unsupervised learning strategies to identify abnormal transaction activity.
- Deep Learning: There are algorithms interspersed with many hidden layers in ML models using neural networks that can study extensive datasets. They then recognize specific behaviors associated with fraudulent activities. These systems remain self-improving and don’t fall behind when new fraud techniques are introduced, like what constantly seems to be happening.
Real-Time Fraud Detection
The most important benefit of AI-based deterrence is the capacity to prevent transactions in areas where fraud is suspected in real-time. Rapid response is one of the defining characteristics of AI systems.
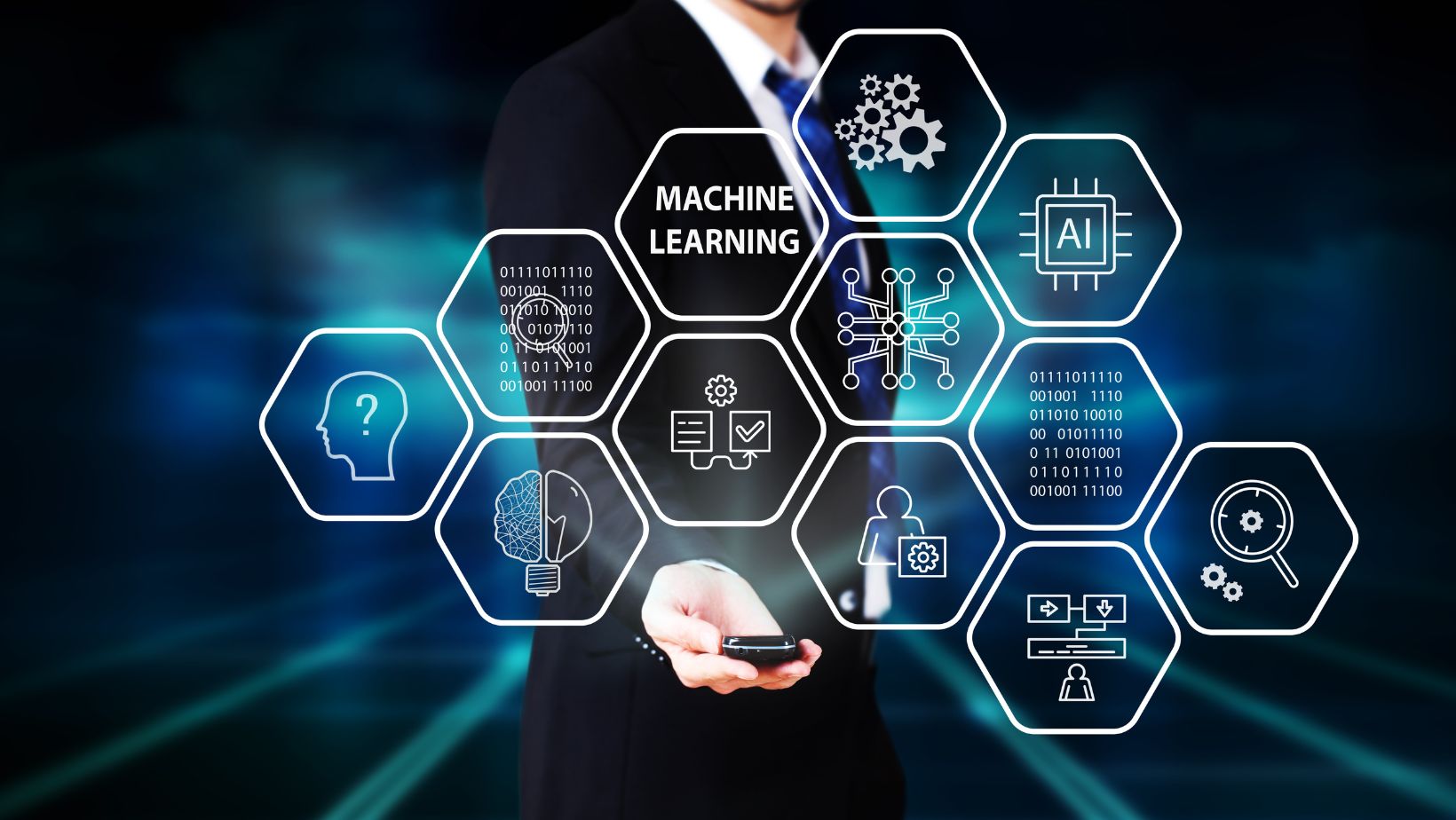
- Behavioral Analytics: AI systems analyze user behavior across several channels with the goal of detecting out-of-the-ordinary acts. An example is eCommerce and an account in an eCommerce platform making several high-value purchases within a short period.
- Natural Language Processing (NLP): NLP assists in the detection of fraud, particularly through analyzing written material, whether in transaction descriptions or interactions with customers. The aim is to identify foul play like phishing or fake account ownership.
- Dynamic Risk Scoring: With AI-based risk scoring, the system assigns risk scores for transactions based on several attributes, including but not limited to geographical location, the device used, and the transaction value. For high-risk activities, clients may have to go through more intense verification processes or have their accounts frozen instantaneously.
Case Studies
There’s so much media coverage about this subject. Here are some real examples:
- Financial Institutions: The Commonwealth Bank of Australia uses AI algorithms to track credit card transactions. As a result, fraud losses were lowered by 40% in a handful of months.
- E-Commerce Platforms: Online merchants are using ML-enhanced systems to determine purchasing trends. For example, one AI model flagged excessive bulk acquisition of gift cards, which turned out to be fraudulent and saved the company possibly millions of dollars. Walmart, Stop & Shop, and Home Depot are the store examples.
- Payment Processors: PayPal is one of the many companies that incorporate ML techniques by reviewing billions of transactions. These kinds of systems detect and suppress fraudulent acts with the utmost precision.
Overcoming Challenges in AI Fraud Detection
Overall, there is strong potential for fraud detection systems based on AI and ML, but there are challenges that need to be addressed:
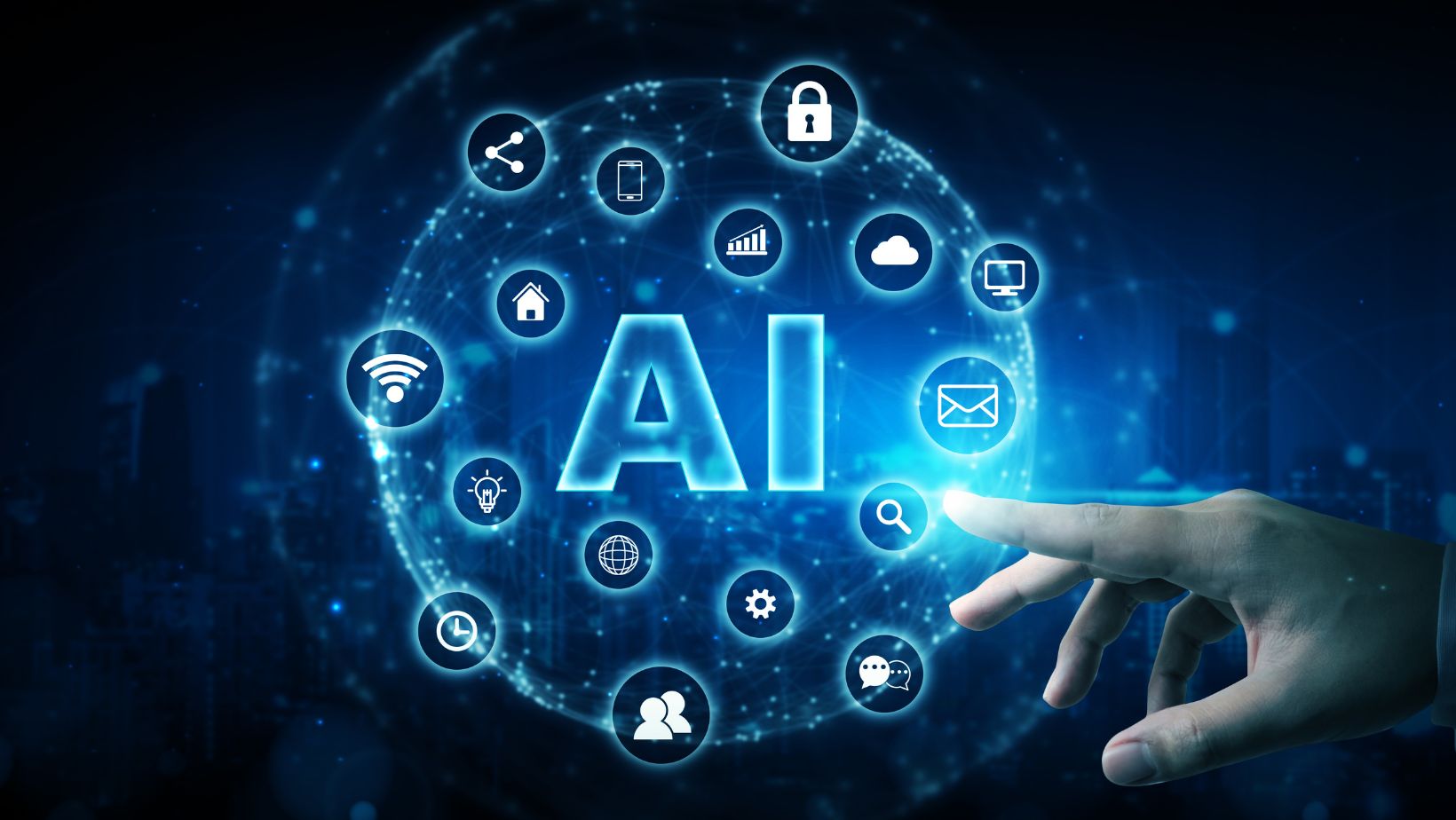
- Data Quality: ML models will always suffer from data that is of low quality or incompleteness. In this case, businesses need to pay attention to building datasets that are representative and updated on a regular basis.
- Integration Complexity: Introducing AI systems into payment ecosystems already in place requires a high level of planning. Organizations need to undertake systemic integration strategies that prevent disruption.
- Regulatory Compliance: Another challenge faced by AI-powered systems is that they must comply with fraud detection systems and data laws like GDPR or CCPA. Compliance with such requirements in the framework of big data analysis is still highly difficult.
With planning and enlisting the expertise of suitable partners, businesses can help achieve the goals of AI in the struggles with fraud.
Many businesses have yet to fully comprehend the transformation AI and ML bring to the industry for payment fraud detection, not only in the US but globally. There’s a massive challenge of protecting customers and their businesses and retaining trust. Soon, however, it is AI and ML that will take the front position in payment fraud management.